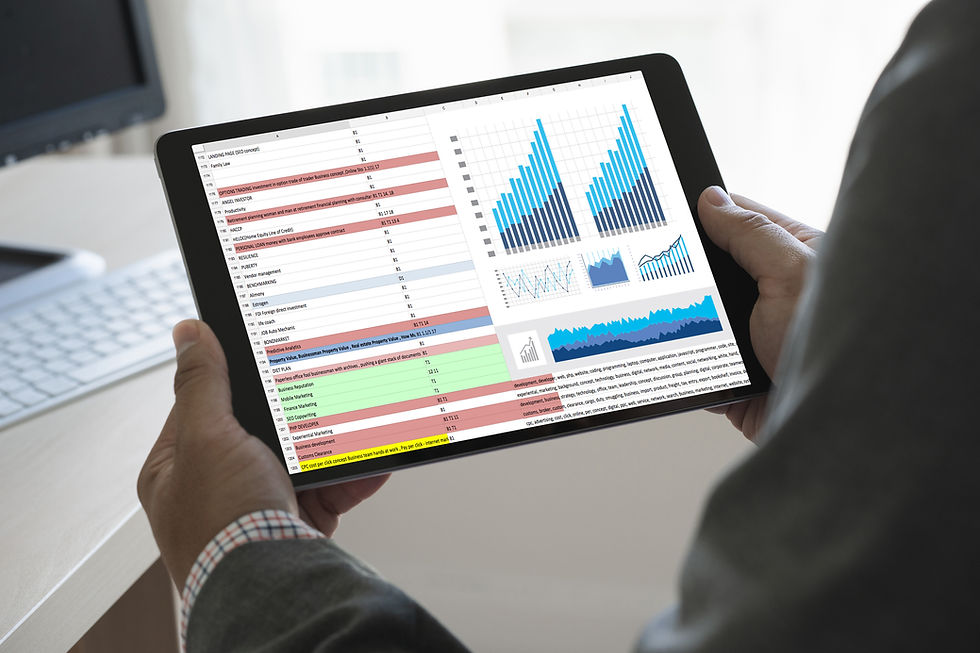
Analytics is hardly new to retail. But with each improvement in analytic processes, the importance of analytical results becomes greater and more ingrained in a retailer’s processes. Now, with the explosion of AI-based analytics that promise to solve some of the trickiest challenges retailers face, the importance of good analytical results is greater than ever.
To ensure reliable results in any analytics system, and particularly in AI-based systems, an organization must get in the habit of managing the inputs, outputs, and integration of results. There are key ‘habits’ that need to be organizationally integrated to gain the benefits of these new tools.
Data hygiene
Over-reliance on analytical results
Tying analytics to business goals
Leaving room for expertise
Precisely defining success
Matching the right analytic approach to the analytic problem
Redesign processes to integrate analytics
1. Data hygiene is more of a data-lifestyle
A weakness of analytical systems is their high sensitivity to the quality and application of data to solving problems. Poor data, be it missing, inaccurate, poorly defined, and/or mismatched, leads to inferior and misleading results. Investment in data for most leading retailers has become fundamental. Some best practice steps:
Precisely define data elements and document(!) these definitions.
Determine a basic atomic level of data collection – maybe its product location, maybe its transaction or T-Log, or other.
Provide attribution of data sufficient to allow synchronization across time periods and across comparable activities.
Remove data islands. Do not store key data in isolated systems that have their own data definitions or limited sync processes.
2. Overreliance on analytical results is probably more of a human habit than a system problem.
Humans don’t think in terms of probabilities very well. We tend to ascribe a certainty of an analytic result that neither the data nor the analysis deserves.
Understand the limits of precision of the data being consumed. If the input data is 40% accurate, it is very hard for the output data to be any better.
Understand the cumulative effect of multiple probabilistic results being brought together. A forecast for sales might have a 20% variation and an independent forecast for inventory might also have a 20% variation but the resulting sell-through forecast, using the two inputs could as much as double the error rate to a 40% error.
Recognize that some results are simply less accurate than others: Consumer demand may be highly variable thus harder to predict accurately whereas inventory might be largely constrained by logistics and limits. Analysis results using both are only as good as the least accurate results – in this case sales.
Interpretation of results: A good analogy is weather: when the average temperature for June 1st is 72 degrees – what does that mean? Does it mean California-like weather where we can expect that the temperature will most likely be 72 degrees? Or will it be more like Chicago where we can expect that temperature to be somewhere between 52 and 92 degrees which average to 72 but it’s no more likely to be 72 degrees than to be 62 or 82.
3. Tying analytics to business goals.
There is certainly plenty to learn from a business as complex as retail, but knowledge for knowledge’s sake is not a good goal for analytics. In fact, by not framing the question precisely analytic results can at best be irrelevant and at worst be misleading.
Take a real-life simple example of an analytics evaluation of e-commerce results with the goal of building out a predictive model for e-commerce sales to guide buying patterns for overall growth. Modeling was predictive in showing strong growth but what wasn’t shown was whether the growth was incremental. The e-commerce growth was almost all cannibalizing store sales – at lower margins. A small twist on the analytics goal to derive whether the incremental sales pattern completely changed the picture.
Time and thought on what really matters to the business is critical to getting the type of results desired.
4. Leaving room for expertise.
Sometimes there is not an effective way to model a problem or sometimes the data does not exist to derive a solution from. Sometimes, the business is such that judgment calls are more predictive than numbers. Expertise and human instincts may be hard to quantify but can be critical to the evaluation and interpretation of analytic results. It is even better to build a mixed-use model that can take in the accumulated knowledge of the experts in the business.
5. Precisely defining success is a combination of many of the other factors discussed.
Analysis is not a one-and-done process. Most analytics builds on itself which requires each piece to be evaluated for success. But how to measure success? The answer is more easily stated than accomplished: as simply and accurately as possible. This takes the real skill of a data scientist and a business user – what exactly is being measured and how to define it? For example, is it more important to define the success of pricing analytics as more accurate forecasting or more return on investment (ROI) on invested inventory? While forecasts are a key ingredient in price determination, there are lots of other factors. Define system success as increasing return on investment via pricing adjustments that will drive the bottom-line results. Defining pricing success as better forecasts might not be at all closely related to better pricing results.
6. Matching up the analytic approach to the analytic problem.
The one-size-fits-all analytics solution is an attractive pitch but don’t succumb to it. Especially with the new AI-based tools available, it has become more important than ever to apply expertise to what methods are used.
AI provides a great example of this. AI-driven analysis is a process that thrives in conditions of massive yet poorly organized and unconnected data. This is the case with many real-world problems but not all. Many times, analytics is performed with well-structured defined data and more traditional optimization and modeling will produce significantly better accuracy and recommendations. An AI model applied to well-regulated sales patterns will not be as good.
Like any tool, it is only best for certain jobs.
7. Redesigning processes to integrate analytics.
Analytics projects usually start small and, unfortunately, many times never evolve beyond a trial stage. One of the most common results of analytic initiatives is not failure but, instead, becoming a ‘science-project.’ An interesting experiment that always seems to sit just outside the day-to-day operations of the business.
To be effective, analytics needs to be used, and to be used it needs to be integrated. For example, a major national retailer had invested millions in a dynamic pricing system with almost real-time pricing capabilities. This was to replace a pricing process that required a 6-week lead time between a price change decision and execution in-store. The benefits of the fast reaction time with sophisticated analytics powering detailed pricing were proven many times across many areas of the business with margin results in the 3 to 5% improvement range – literally 100’s of millions of dollars. Yet, the 6-week lead time process step was never removed. This essentially eliminated the benefit of the analytic pricing solutions – not because it failed but because the simple process step change was never made.
In short, it is all too common to miss out on the promise of analytics to improve your business by skipping the habits that need to form to support these solutions. As the power of analytics evolves to tackle more problems and the benefit cases get stronger it is mandatory that successful businesses prepare themselves to take advantage of these new tools by building the institutional best practices to support them.
To harness the full power of AI analytics, retailers must develop these seven key habits. By prioritizing data hygiene, avoiding overreliance on analytics, aligning analytic efforts with business goals, valuing human expertise, choosing the right approaches, redesigning processes, and building strong organizational practices, retailers can unlock new levels of efficiency, customer satisfaction, and business performance.