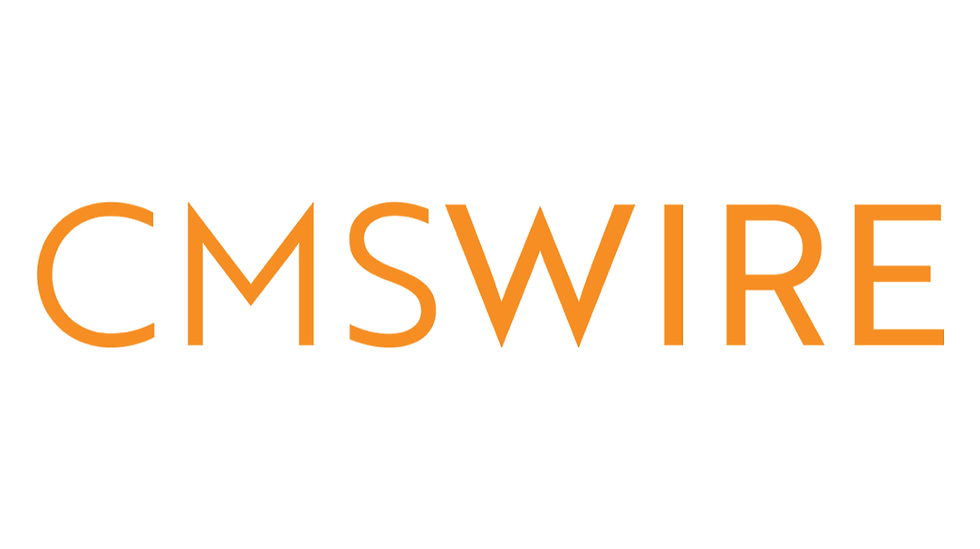
Find the original article in CMSWire here.
Unify, clean and automate your data science lifecycle. Here's how.
The Gist
Speed matters most. Real-time insights give companies a competitive edge by turning data into action faster.
Unify and automate. Centralized data and automation shrink the data science lifecycle from months to minutes.
Visibility drives trust. End-to-end transparency empowers teams to make better decisions and maintain clean data.
Data is the lifeblood of business operations. Whether companies rely on monolithic platforms or integrated solutions, success hinges on how quickly data moves through the organization. Yet, for many, the data science lifecycle still takes months, which delays critical decisions and stalls progress.
To compete in real-time markets, companies need more than faster data; they need clean, actionable insights delivered instantly. When business users can access reliable data within minutes, they can make smarter decisions, respond to trends and drive growth.
The Chief Data Officer (CDO) and Chief Customer Officer (CCO) play a pivotal role in this transformation. The CDO ensures data is not only fast but also accurate, standardized and governed for enterprise-wide usability. Meanwhile, the CCO depends on real-time, high-quality insights to enhance customer experiences, personalize interactions and anticipate needs. When these leaders align, they can bridge the gap between raw data and strategic action, ensuring that the organization leverages data science for immediate, meaningful impact.
While every company wants faster, cleaner data, traditional processes can be complex and bog down the lifecycle. Accelerating data science happens when companies embrace automation, unified data and end-to-end visibility, which allows them to tap into real-time insights.
Slow and Siloed: The Traditional Data Science Lifecycle
For most companies, the typical data science lifecycle can take up to half a year or more to complete, as data flows through a complex, multi-step process. The data funnels through multiple departments and steps.
Here’s what a traditional process includes:
Data science creation: PhDs on the data science team develop algorithms based on business needs. For instance, a fashion retailer may want to improve how their merchandising team optimizes product pricing, so the data scientists build the algorithm.
Solution development: Coders translate algorithms into solutions. In the case of a fashion retailer, this could be a solution that optimizes pricing, for example.
User testing: End users take the developed solution and test the models, dashboards and results. At our hypothetical retailer, this means the merchandising team tests the price optimization tool and takes notes on the intuitiveness of the dashboard or how the results are aggregated.
Iteration: If the model falls short during testing, the cycle starts again. Data scientists go back to retooling the model.
Depending on how many iterations a model goes through, a traditional data science lifecycle can go on for a very long time. The back-and-forth between teams can take months. For the fashion retailer, that’s long enough that seasonal opportunities can be missed.
In healthcare, the stakes are even higher. Patient data must be processed, cleaned and analyzed quickly to support timely care. Whether it's pricing, patient outcomes or supply chain decisions, months-long data cycles no longer meet business needs.
How to Shorten the Data Lifecycle
To shrink the data lifecycle from months to minutes, companies need to rethink their approach and focus on cleaning and automating data to attain real-time insights. Business leaders have been achieving these massive time savings by following these key steps:
Unifying data: Creating one single source of truth for data is paramount. To control the data lifecycle, companies first need to make sure that all their data (inside and outside the company) funnels to one centralized location. This creates a single source of truth, where data scientists and business users ultimately work with the best data available.
Learning Opportunities
Cleaning data with AI: As data gets funneled to a centralized location, AI can clean the data, properly format it and standardize it for seamless integration.
Automating the processing: Loading data into solutions automatically results in faster data processing and makes it immediately available for use.
Governing the data: It’s not enough to let AI clean data. Companies must educate business users on best practices when it comes to handling data, especially unified data living inside a central location. For instance, business users should never remove data from the system and reupload it. The data instantly becomes unclean. Clean data fuels AI; poor data undermines it.
Implementing end-to-end visibility: Lastly, as data flows across business users and within different solutions, it’s important that all stakeholders, including C-suite executives, can see how the data flows through the company’s platform.
As the data science lifecycle continues to update in real time at an automated and accelerated pace, the data needs to be monitored and seen by all stakeholders. This process greatly enhances how companies use data, develop solutions and optimize their businesses. In the case of the fashion retailer, rather than wait for a price optimization solution to go through various iterations, the merchandising business user can access visible pricing data and see immediate impacts on the business.
Core Questions Around the Data Science Lifecycle
Editor's note: Here are two important questions to ask about the data lifecycle:
How can companies accelerate the data science lifecycle?
Companies can accelerate it by unifying data into a single source of truth, using AI to clean and standardize data, automating workflows and implementing end-to-end visibility across teams and systems.
What are the biggest roadblocks to speeding up your lifecycle?
The biggest roadblocks include siloed data across departments, manual data cleaning processes, lack of automation and poor visibility into how data moves through the organization.